Graphic Cards
NVIDIA A100 Aces Throughput, Latency Results in Key Inference Benchmark for Financial Services Industry
NVIDIA A100 Tensor Core GPUs operating on Supermicro servers have captured main outcomes for inference within the newest STAC-ML Markets benchmark, a key expertise efficiency gauge for the monetary companies trade.
The outcomes present NVIDIA demonstrating unequalled throughput — serving up hundreds of inferences per second on probably the most demanding fashions — and prime latency on the newest STAC-ML inference normal.
The outcomes are intently adopted by monetary establishments, three-quarters of which depend on machine studying, deep studying or excessive efficiency computing, in accordance with a latest survey.
NVIDIA A100: Prime Latency Outcomes
The STAC-ML inference benchmark is designed to measure the latency of lengthy short-term reminiscence (LSTM) mannequin inference — the time from receiving new enter knowledge till the mannequin output is computed. LSTM is a key mannequin method used to find monetary time-series knowledge like asset costs.
The benchmark consists of three LSTM fashions of accelerating complexity. NVIDIA A100 GPUs, operating in a Supermicro Extremely SuperServer, demonstrated low latencies within the 99th percentile.
Accelerated Computing for STAC-ML and STAC-A2, STAC-A3 Benchmarks
Contemplating the A100 efficiency on STAC-ML for inference — along with its record-setting efficiency within the STAC-A2 benchmark for choice value discovery and the STAC-A3 benchmark for mannequin backtesting — supplies a glimpse at how NVIDIA AI computing can speed up a pipeline of contemporary buying and selling environments.
It additionally exhibits A100 GPUs ship main efficiency and workload versatility for monetary establishments.
Predictable Efficiency for Constant Low Latency
Predictable efficiency is essential for low-latency environments in finance, as excessive outliers may cause substantial losses throughout quick market strikes.
Notably, there have been no massive outliers in NVIDIA’s latency, as the utmost latency was not more than 2.3x the median latency throughout all LSTMs and the variety of mannequin situations, ranging as much as 32 concurrent situations.1
NVIDIA is the primary to submit efficiency outcomes for what’s referred to as the Tacana Suite of the benchmark. Tacana is for inference carried out on a sliding window, the place a brand new timestep is added and the oldest eliminated for every inference operation. That is useful for high-frequency buying and selling, the place inference must be carried out on each market knowledge replace.
A second suite, Sumaco, performs inference on a completely new set of knowledge, which displays the use case the place an occasion prompts inference based mostly on latest historical past.
Main Throughput in Benchmark Outcomes
NVIDIA additionally submitted a throughput-optimized configuration on the identical {hardware} for the Sumaco Suite in FP16 precision.2
On the least complicated LSTM within the benchmark, A100 GPUs on Supermicro servers helped serve up greater than 1.7 million inferences per second.3
For probably the most complicated LSTM, these methods dealt with as many as 12,800 inferences per second.4
NVIDIA A100: Efficiency and Versatility
NVIDIA GPUs supply a number of benefits that decrease the overall value of possession for digital buying and selling stacks.
For one, NVIDIA AI supplies a single platform for coaching and inference. Whether or not creating, backtesting or deploying an AI mannequin, NVIDIA AI delivers main efficiency — and builders don’t have to be taught totally different programming languages and frameworks for analysis and buying and selling.
Furthermore, the NVIDIA CUDA programming mannequin permits improvement, optimization and deployment of purposes throughout GPU-accelerated embedded methods, desktop workstations, enterprise knowledge facilities, cloud-based platforms and HPC supercomputers.
Efficiencies for Decreased Working Bills
The monetary companies trade stands to learn from not solely knowledge throughput advances but in addition improved operational efficiencies.
Decreased vitality and sq. footage utilization for methods in knowledge facilities could make an enormous distinction in working bills. That’s particularly urgent as IT organizations make the case for budgetary outlays to cowl new high-performance methods.
On probably the most demanding LSTM mannequin, NVIDIA A100 exceeded 17,700 inferences per second per kilowatt whereas consuming 722 watts, providing main vitality effectivity.5
The benchmark outcomes verify that NVIDIA GPUs are unequalled by way of throughput and vitality effectivity for workloads like backtesting and simulation.
Find out about NVIDIA delivering smarter, safer monetary companies.
[1] SUT ID NVDA221118b, max of STAC-ML.Markets.Inf.T.LSTM_A.2.LAT.v1
[2] SUT ID NVDA221118a
[3] STAC-ML.Markets.Inf.S.LSTM_A.4.TPUT.v1
[4] STAC-ML.Markets.Inf.S.LSTM_C.[1,2,4].TPUT.v1
[5] SUT ID NVDA221118a, STAC-ML.Markets.Inf.S.LSTM_C.[1,2,4].ENERG_EFF.v1
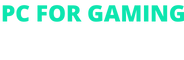